1. Introduction
Objective
This study aimed to show that immunosuppressive treatment with tocilizumab was more effective than steroid therapy alone, and positive results for secondary infections did not affect the patient’s prognosis, which indicated the therapy’s safety.
2. Materials and Methods
Steroid therapy was initiated on Day 0 for all patients meeting ARDS criteria. The starting dose was adjusted according to ARDS severity, with mild cases receiving 6 mg/day of dexamethasone, moderate cases 10 mg/day, and severe cases 20 mg/day. However, doses could be escalated or adjusted dynamically within individual patients, depending on clinical progression, oxygenation status (PaO2/FiO2), and radiological findings. The therapy was continued for a maximum of 14 days or until significant clinical improvement was observed, whichever came first.
Tocilizumab therapy was reserved for patients with severe COVID-19 pneumonia, defined by PaO2/FiO2 < 300 and progressive lesions on HRCT. It was administered intravenously at a dose of 8 mg/kg (maximum 800 mg) as a single dose, typically within the first 48 h of ICU admission. No additional doses were given, even in cases of limited initial response. Exclusion criteria for tocilizumab included coexisting infections, PaO2/FiO2 > 300, non-progressive fibrotic lesions on HRCT, and severe organ dysfunction or hematologic disorders. All patients received standardized peri-COVID care, regardless of therapy type. Blood cultures were obtained every 72 h, and bronchoalveolar lavage (BAL) samples were collected every 48 h to monitor secondary infections. Cultures with bacterial concentrations > 105 CFU/mL or clinical symptoms of infection were treated with appropriate antibiotics. Patients with positive culture results were classified into the study group, and patients with negative results were included in the control group. Baseline characteristics, including age, gender, and key comorbidities, were incorporated into the multivariable Cox proportional hazards model to assess factors influencing survival. Vaccination status was not included as a variable in the model, as all patients in this study were unvaccinated due to the inclusion criteria. Hence, this analysis focused on relevant factors without redundancy. The primary endpoint was survival until ICU discharge. Details of the statistical methods and adjustments for confounding variables are provided in the Statistics Section. This study adhered to the Helsinki Declaration and was approved by the Bioethics Commission at the Regional Medical Chamber in Zielona Góra, Poland (No. 21/157/2021).
Statistics
The statistical methods used included logistic regression with sub-model selection based on the Akaike information criterion (AIC) to model the probability of patient survival. Kaplan–Meier survival curves and the log-rank test were used to assess differences in survival function according to the selected variables. To adjust for potential confounding factors, such as age, gender, comorbidities, and concurrent medications, multivariable logistic regression analyses and a Cox proportional hazard model were employed, allowing for the evaluation of the association between treatment strategies and survival outcomes while accounting for key baseline characteristics. Pearson’s correlation coefficient, the chi-square test, Fisher’s exact test, and Student’s t-test were used to assess relationships between variables. The distributions of variables are presented using box plots for quantitative variables and bar charts for discrete variables. All statistical analyses were performed with statistical significance set at p < 0.05.
3. Results
All patients included in this study were unvaccinated due to inclusion criteria, either by personal choice or due to medical contraindications; hence, the vaccination status was not included as a variable in the model. Inflammatory indicators: PCT—procalcitonin assay, CRP—C reactive protein, NEU—neutrophil, PLT—platelet; Comorbidities: AH—arterial hypertension, AF—atrial fibrillation, CHD—coronary heart disease, HF—heart failure, CKD—chronic kidney disease, COPD—chronic obstructive pulmonary disease, DM2—diabetes mellitus 2; Secondary infections: NDM—New Delhi metallo-β-lactamase, MRSA—Methicillin-resistant Staphylococcus aureus, GRE—Glycopeptide-resistant Enterococcus, VRE—Vancomycin-resistant Enterococci.
4. Discussion
The key finding of our research is that tocilizumab appeared to be indirectly connected to some potential secondary bacterial infections while also contributing to the decreased mortality rate through its immunomodulatory effects. To understand this multidimensional problem, basic immunologic mechanisms and the phenomenon of cytokine storms in COVID-19 should be accounted for.
4.1. Immunologic Mechanisms in COVID-19 and the Cytokine Storm
4.2. A Perfect Support or a Main Player?
4.3. Tocilizumab and ICU Admission Criteria
4.4. Tocilizumab and Age
4.5. Tocilizumab and Secondary Infections
4.6. Comorbidities
4.7. Study Limitations
The main limitation of our study is the heterogeneity of the patients included in the analyses. This was a single-center study, with a limited sample size, which may restrict the generalizability of the findings. The retrospectively collected data are potentially connected with inconsistences or gaps in the information (25 patients were excluded due to incomplete data). Furthermore, observational studies can potentially be influenced by confounding factors such as unmeasured variables or selection bias.
5. Conclusions
Our findings support the hypothesis that therapy based on tocilizumab and steroids is associated with improved prognosis in patients with severe COVID-19-related ARDS treated in the Intensive Care Unit. However, due to this study’s observational nature, further controlled trials are needed to confirm causality and refine therapeutic recommendations.
The use of tocilizumab was observed to decrease the mortality rate in critically ill patients with severe COVID-19-related ARDS who qualified for ICU procedures and MV.
Suppression of the cytokine storm appears to be crucial in the survival of patients with severe COVID-19-related ARDS. This interpretation is supported by the well-documented mechanisms of IL-6 blockade with tocilizumab and the observed trends in our study.
As an immunomodulating agent, tocilizumab was a safe treatment option in severe COVID-19 infection.
The increased risk for positive cultures of pathogens potentially responsible for secondary infections was the side effect of tocilizumab administration.
The positive cultures of pathogens potentially responsible for secondary infections were not connected with the ICU patient survival rate.
Take Home Message:
Tocilizumab is a safe immunomodulatory drug that can limit the frequency and severity of the cytokine storm in infections—in this case, SARS-CoV-2. Its effect on modulating and limiting the inflammatory response may increase the risk of secondary infections, but this phenomenon does not affect the mortality of patients in the ICU.
Author Contributions
W.K. and J.Z.: data analysis and manuscript writing. J.K., M.M., M.S., D.Z., A.N. and P.N.: data collection and database creation. B.K.: content supervision and manuscript editing. All authors have read and agreed to the published version of the manuscript.
Funding
This research received no external funding.
Institutional Review Board Statement
The study was conducted in accordance with the Declaration of Helsinki, and approved by the Institutional Ethics Committee of the Regional Medical Chamber in Zielona Góra, Poland (No. 21/157/2021) for studies involving humans.
Informed Consent Statement
Informed consent was obtained from all subjects involved in the study.
Data Availability Statement
The data that support the findings of this study are available upon request from the corresponding author [Wiktoria Kulińska].
Acknowledgments
Thanks to Ilona Kauf for her support and commitment.
Conflicts of Interest
The authors declare no conflicts of interest.
References
- World Health Organization 2023 Data.who.int, WHO Coronavirus (COVID-19) Dashboard. Available online: https://data.who.int/dashboards/covid19/cases (accessed on 5 November 2024).
- Bohn, M.K.; Hall, A.; Sepiashvili, L.; Jung, B.; Steele, S.; Adeli, K. Pathophysiology of COVID-19: Mechanisms Underlying Disease Severity and Progression. Physiology 2020, 35, 288–301. [Google Scholar] [CrossRef] [PubMed] [PubMed Central]
- Wang, J.; Yang, X.; Li, Y.; Huang, J.A.; Jiang, J.; Su, N. Specific cytokines in the inflammatory cytokine storm of patients with COVID-19-associated acute respiratory distress syndrome and extrapulmonary multiple-organ dysfunction. Virol. J. 2021, 18, 117. [Google Scholar] [CrossRef] [PubMed] [PubMed Central]
- Leisman, D.E.; Ronner, L.; Pinotti, R.; Taylor, M.D.; Sinha, P.; Calfee, C.S.; Hirayama, A.V.; Mastroiani, F.; Turtle, C.J.; Harhay, M.O.; et al. Cytokine elevation in severe and critical COVID-19: A rapid systematic review, meta-analysis, and comparison with other inflammatory syndromes. Lancet Respir. Med. 2020, 8, 1233–1244. [Google Scholar] [CrossRef] [PubMed] [PubMed Central]
- Bime, C.; Casanova, N.; Oita, R.C.; Ndukum, J.; Lynn, H.; Camp, S.M.; Lussier, Y.; Abraham, I.; Carter, D.; Miller, E.J.; et al. Development of a biomarker mortality risk model in acute respiratory distress syndrome. Crit. Care 2019, 23, 410. [Google Scholar] [CrossRef] [PubMed] [PubMed Central]
- McElvaney, O.J.; Curley, G.F.; Rose-John, S.; McElvaney, N.G. Interleukin-6: Obstacles to targeting a complex cytokine in critical illness. Lancet Respir. Med. 2021, 9, 643–654. [Google Scholar] [CrossRef] [PubMed] [PubMed Central]
- Ranieri, V.M.; Rubenfeld, G.D.; Thompson, B.T.; Ferguson, N.D.; Caldwell, E.; Fan, E.; Camporota, L.; Slutsky, A.S. Acute respiratory distress syndrome: The Berlin Definition. JAMA 2012, 307, 2526–2533. [Google Scholar] [CrossRef] [PubMed]
- Villar, J.; Pérez-Méndez, L.; Blanco, J.; Añón, J.M.; Blanch, L.; Belda, J.; Santos-Bouza, A.; Fernández, R.L.; Kacmarek, R.M.; Spanish Initiative for Epidemiology, Stratification, and Therapies for ARDS (SIESTA) Network. A universal definition of ARDS: The PaO2/FiO2 ratio under a standard ventilatory setting–a prospective, multicenter validation study. Intensive Care Med. 2013, 39, 583–592. [Google Scholar] [CrossRef] [PubMed]
- Vincent, J.L.; Slutsky, A.S. We’ve never seen a patient with ARDS! Intensive Care Med. 2020, 46, 2133–2135. [Google Scholar] [CrossRef] [PubMed]
- Tsai, P.H.; Lai, W.Y.; Lin, Y.Y.; Luo, Y.H.; Lin, Y.T.; Chen, H.K.; Chen, Y.M.; Lai, Y.C.; Kuo, L.C.; Chen, S.D.; et al. Clinical manifestation and disease progression in COVID-19 infection. J. Chin. Med. Assoc. 2021, 4, 3–8. [Google Scholar] [CrossRef] [PubMed]
- Tajbakhsh, A.; Gheibi Hayat, S.M.; Taghizadeh, H.; Akbari, A.; Inabadi, M.; Savardashtaki, A.; Johnston, T.P.; Sahebkar, A. COVID-19 and cardiac injury: Clinical manifestations, biomarkers, mechanisms, diagnosis, treatment, and follow up. Expert Rev. Anti Infect. Ther. 2021, 19, 345–357. [Google Scholar] [CrossRef] [PubMed]
- Beyerstedt, S.; Casaro, E.B.; Rangel, É.B. COVID-19: Angiotensin-converting enzyme 2 (ACE2) expression and tissue susceptibility to SARS-CoV-2 infection. Eur. J. Clin. Microbiol. Infect. Dis. 2021, 40, 905–919. [Google Scholar] [CrossRef] [PubMed] [PubMed Central]
- Cantuti-Castelvetri, L.; Ojha, R.; Pedro, L.D.; Djannatian, M.; Franz, J.; Kuivanen, S.; van der Meer, F.; Kallio, K.; Kaya, T.; Anastasina, M.; et al. Neuropilin-1 facilitates SARS-CoV-2 cell entry and infectivity. Science 2020, 370, 856–860. [Google Scholar] [CrossRef] [PubMed] [PubMed Central]
- Mayi, B.S.; Leibowitz, J.A.; Woods, A.T.; Ammon, K.A.; Liu, A.E.; Raja, A. The role of Neuropilin-1 in COVID-19. PLoS Pathog. 2021, 17, e1009153. [Google Scholar] [CrossRef] [PubMed] [PubMed Central]
- Ashraf, U.M.; Abokor, A.A.; Edwards, J.M.; Waigi, E.W.; Royfman, R.S.; Hasan, S.A.; Smedlund, K.B.; Hardy, A.M.; Chakravarti, R.; Koch, L.G. SARS-CoV-2, ACE2 expression, and systemic organ invasion. Physiol. Genom. 2021, 53, 51–60. [Google Scholar] [CrossRef] [PubMed] [PubMed Central]
- Parasher, A. COVID-19: Current understanding of its Pathophysiology, Clinical presentation and Treatment. Postgrad Med. J. 2021, 97, 312–320. [Google Scholar] [CrossRef] [PubMed] [PubMed Central]
- Zanza, C.; Romenskaya, T.; Manetti, A.C.; Franceschi, F.; La Russa, R.; Bertozzi, G.; Maiese, A.; Savioli, G.; Volonnino, G.; Longhitano, Y. Cytokine Storm in COVID-19: Immunopathogenesis and Therapy. Medicina 2022, 58, 144. [Google Scholar] [CrossRef] [PubMed] [PubMed Central]
- Queiroz, M.A.F.; Neves, P.F.M.D.; Lima, S.S.; Lopes, J.D.C.; Torres, M.K.D.S.; Vallinoto, I.M.V.C.; Bichara, C.D.; Santos, E.F.; de Brito, M.T.; da Silva, A.L.; et al. Cytokine Profiles Associated With Acute COVID-19 and Long COVID-19 Syndrome. Front. Cell Infect. Microbiol. 2022, 12, 922422. [Google Scholar] [CrossRef] [PubMed] [PubMed Central]
- Coperchini, F.; Chiovato, L.; Ricci, G.; Croce, L.; Magri, F.; Rotondi, M. The cytokine storm in COVID-19: Further advances in our understanding the role of specific chemokines involved. Cytokine Growth Factor Rev. 2021, 58, 82–91. [Google Scholar] [CrossRef] [PubMed] [PubMed Central]
- Alahyari, S.; Rajaeinejad, M.; Jalaeikhoo, H.; Amani, D. Regulatory T Cells in Immunopathogenesis and Severity of COVID-19: A Systematic Review. Arch. Iran. Med. 2022, 25, 127–132. [Google Scholar] [CrossRef] [PubMed]
- Avni, T.; Leibovici, L.; Cohen, I.; Atamna, A.; Guz, D.; Paul, M.; Gafter-Gvili, A.; Yahav, D. Tocilizumab in the treatment of COVID-19-a meta-analysis. QJM 2021, 114, 577–586. [Google Scholar] [CrossRef] [PubMed] [PubMed Central]
- RECOVERY Collaborative Group. Dexamethasone in hospitalized patients with COVID-19. N. Engl. J. Med. 2021, 384, 693–704. [Google Scholar] [CrossRef]
- Narasaraju, T.; Ravi, Y. High-dose corticosteroid therapy in COVID-19: The RECOVERY trial [Letter to the Editor]. Lancet 2024, 403, 1338–1339. [Google Scholar] [CrossRef]
- WHO Rapid Evidence Appraisal for COVID-19 Therapies (REACT) Working Group. Association between administration of systemic corticosteroids and mortality among critically ill patients with COVID-19: Meta-analysis. JAMA 2020, 324, 1330–1341. [Google Scholar] [CrossRef] [PubMed]
- Gulick, R.M.; Pau, A.K.; Daar, E.; Evans, L.; Gandhi, R.T.; Tebas, P.; Ridzon, R.; Masur, H.; Lane, H.C.; NIH COVID-19 Treatment Guidelines Panel; et al. National Institutes of Health COVID-19 Treatment Guidelines Panel: Perspectives and Lessons Learned. Ann. Intern. Med. 2024, 177, 1547–1557. [Google Scholar] [CrossRef] [PubMed]
- Salton, F.; Confalonieri, P.; Meduri, G.U.; Mondini, L.; Trotta, L.; Barbieri, M.; Bozzi, C.; Torregiani, C.; Lerda, S.; Bellan, M.; et al. Theory and Practice of Glucocorticoids in COVID-19: Getting to the Heart of the Matter—A Critical Review and Viewpoints. Pharmaceuticals 2023, 16, 924. [Google Scholar] [CrossRef]
- Stone, J.H.; Frigault, M.J.; Serling-Boyd, N.J.; Fernandes, A.D.; Harvey, L.; Foulkes, A.S.; Horick, N.K.; Healy, B.C.; Shah, R.; Bensaci, A.M.; et al. Efficacy of Tocilizumab in Patients Hospitalized with COVID-19. N. Engl. J. Med. 2020, 383, 2333–2344. [Google Scholar] [CrossRef] [PubMed]
- Schiff, M.H.; Kremer, J.M.; Jahreis, A.; Vernon, E.; Isaacs, J.D.; van Vollenhoven, R.F. Integrated safety in tocilizumab clinical trials. Arthritis Res. Ther. 2011, 13, R141. [Google Scholar] [CrossRef] [PubMed]
- Campbell, L.; Chen, C.; Bhagat, S.S.; Parker, R.A.; Östör, A.J.K. Risk of adverse events including serious infections in rheumatoid arthritis patients treated with tocilizumab: A systematic literature review and meta-analysis of randomized controlled trials. Rheumatology 2011, 50, 552–562. [Google Scholar] [CrossRef]
- Guaraldi, G.; Meschiari, M.; Cozzi-Lepri, A.; Milic, J.; Tonelli, R.; Menozzi, M.; Franceschini, E.; Cuomo, G.; Orlando, G.; Borghi, V.; et al. Tocilizumab in patients with severe COVID-19: A retrospective cohort study. Lancet Rheumatol. 2020, 2, e474–e484. [Google Scholar] [CrossRef] [PubMed] [PubMed Central]
- Gupta, S.; Wang, W.; Hayek, S.S.; Chan, L.; Mathews, K.S.; Melamed, M.L.; Brenner, S.K.; Leonberg-Yoo, A.; Schenck, E.J.; Radbel, J.; et al. Association Between Early Treatment with Tocilizumab and Mortality Among Critically Ill Patients with COVID-19. JAMA Intern. Med. 2021, 181, 41–51. [Google Scholar] [CrossRef] [PubMed]
- Biran, N.; Ip, A.; Ahn, J.; Go, R.C.; Wang, S.; Mathura, S.; Sinclaire, B.A.; Bednarz, U.; Marafelias, M.; Hansen, E.; et al. Tocilizumab among patients with COVID-19 in the intensive care unit: A multicentre observational study. Lancet Rheumatol. 2020, 2, e603–e612. [Google Scholar] [CrossRef] [PubMed]
The probability of survival of COVID-19 patients in the ICU in relation to sex, age, and therapy described by the Kaplan–Meyer curves: (A) Survival probability by sex, with a trend favoring females, though without sufficient statistical significance. (B) Survival probability by therapy, favoring tocilizumab + steroids with marginal statistical significance (p = 0.051). (C) Survival probability by age, with a trend favoring younger patients, which is statistically significant (p < 0.001). Age (years); types of therapy used: steroids, tocilizumab + steroids.
Figure 1.
The probability of survival of COVID-19 patients in the ICU in relation to sex, age, and therapy described by the Kaplan–Meyer curves: (A) Survival probability by sex, with a trend favoring females, though without sufficient statistical significance. (B) Survival probability by therapy, favoring tocilizumab + steroids with marginal statistical significance (p = 0.051). (C) Survival probability by age, with a trend favoring younger patients, which is statistically significant (p < 0.001). Age (years); types of therapy used: steroids, tocilizumab + steroids.
Age comparison to treatment methods described by the Kaplan–Meyer curves. The differences between the curves are statistically significant (p < 0.05). Regardless of the therapy used, younger patients have a higher survival chance than older ones. Tocilizumab combined with steroids appears to improve outcomes mostly in middle-aged patients (41–60 years), but the effect is less pronounced in the oldest cohort (≥61 years).
Figure 2.
Age comparison to treatment methods described by the Kaplan–Meyer curves. The differences between the curves are statistically significant (p < 0.05). Regardless of the therapy used, younger patients have a higher survival chance than older ones. Tocilizumab combined with steroids appears to improve outcomes mostly in middle-aged patients (41–60 years), but the effect is less pronounced in the oldest cohort (≥61 years).
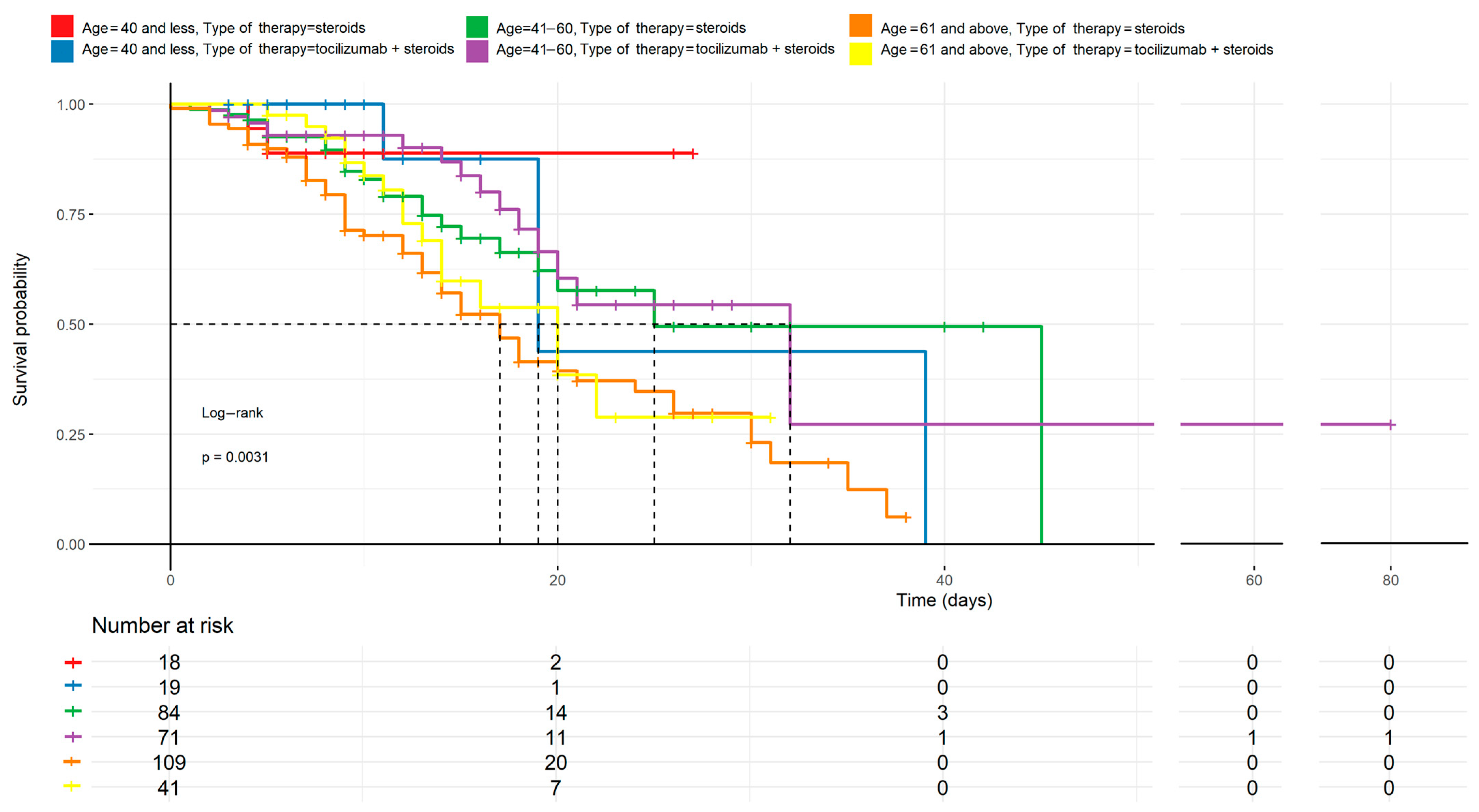
Survival probability of patients with comorbidities such as AF and CHD described by the Kaplan–Meyer curves. The groups of patients suffering from the diseases are smaller in size, though there is a high statistical difference in survival probability favoring patients without CHD (B) and AF (A) in severe COVID-19 (respectively, p = 0.025, p = 0.008). AF—atrial fibrillation, CHD—coronary heart disease.
Figure 3.
Survival probability of patients with comorbidities such as AF and CHD described by the Kaplan–Meyer curves. The groups of patients suffering from the diseases are smaller in size, though there is a high statistical difference in survival probability favoring patients without CHD (B) and AF (A) in severe COVID-19 (respectively, p = 0.025, p = 0.008). AF—atrial fibrillation, CHD—coronary heart disease.
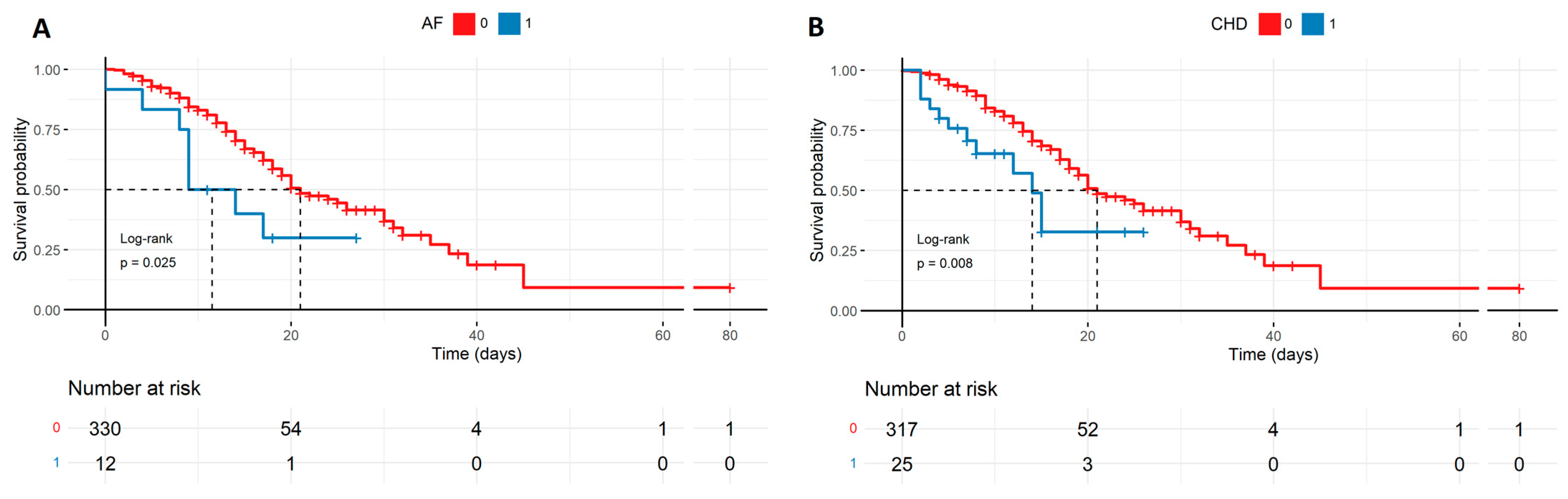
Quality chart of patients showing proportions of age, presence of AF, CHD, and mortality with regard to applied therapy. The therapy including tocilizumab + steroids was more frequently used in patients <40 y.o., without AF and/or CHD. The difference between the therapies (toci + steroids vs. steroids) was greater in patients who died (29.3% vs. 70.7%) compared to patients who survived (42.9% vs. 57.1%). AF—atrial fibrillation, CHD—coronary heart disease.
Figure 4.
Quality chart of patients showing proportions of age, presence of AF, CHD, and mortality with regard to applied therapy. The therapy including tocilizumab + steroids was more frequently used in patients <40 y.o., without AF and/or CHD. The difference between the therapies (toci + steroids vs. steroids) was greater in patients who died (29.3% vs. 70.7%) compared to patients who survived (42.9% vs. 57.1%). AF—atrial fibrillation, CHD—coronary heart disease.
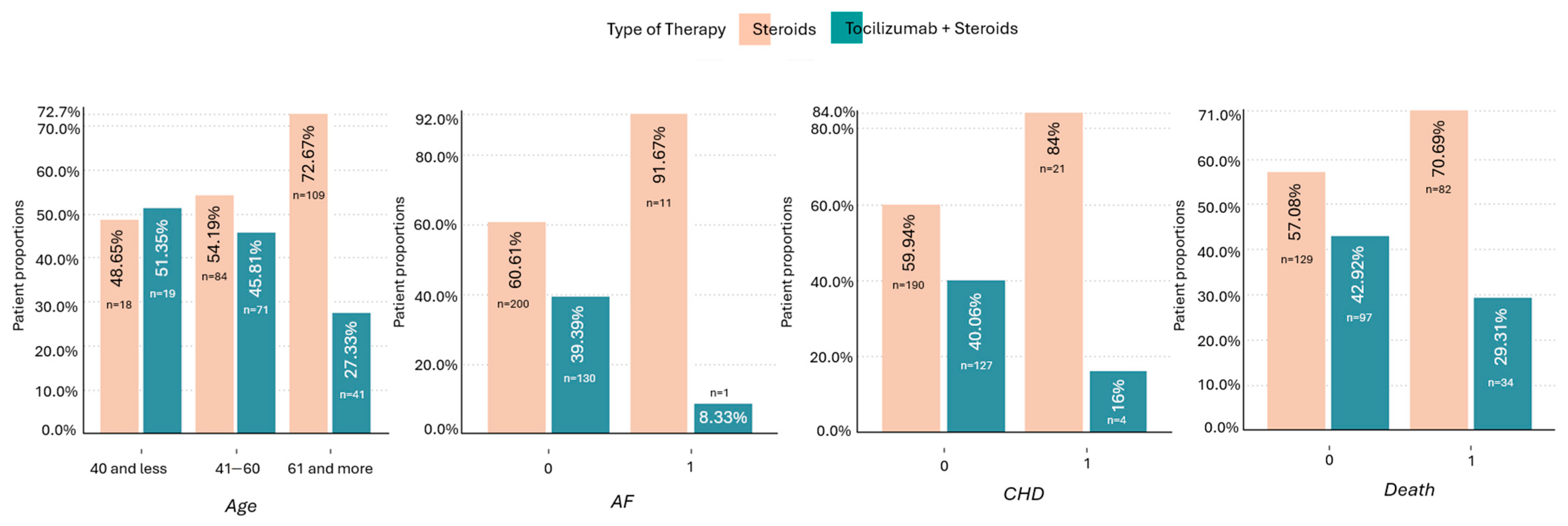
Potential secondary infections in all patients described by the Kaplan–Meyer curves: (A) The survival probability regarding NDM infection favoring patients with NDM, which is statistically significant (p < 0.0001). The statistical significance is marginal (p = 0.051) in the case of A. baumanii (B) with the same trend, though there is no statistical significance in mortality prediction in the case of VRE and GRE (C,D). The patients with NDM are also the most proportional compared to the patients without secondary infection regarding numbers, meaning it was the most popular SI. NDM—Klebsiella pneumoniae New Delhi metallo-β-lactamase-resistant, VRE—Vancomycin-resistant Enterococcus, A. baumanii—Acinetobacter baumanii, and GRE—Glycopeptide-resistant Enterococcus.
Figure 5.
Potential secondary infections in all patients described by the Kaplan–Meyer curves: (A) The survival probability regarding NDM infection favoring patients with NDM, which is statistically significant (p < 0.0001). The statistical significance is marginal (p = 0.051) in the case of A. baumanii (B) with the same trend, though there is no statistical significance in mortality prediction in the case of VRE and GRE (C,D). The patients with NDM are also the most proportional compared to the patients without secondary infection regarding numbers, meaning it was the most popular SI. NDM—Klebsiella pneumoniae New Delhi metallo-β-lactamase-resistant, VRE—Vancomycin-resistant Enterococcus, A. baumanii—Acinetobacter baumanii, and GRE—Glycopeptide-resistant Enterococcus.
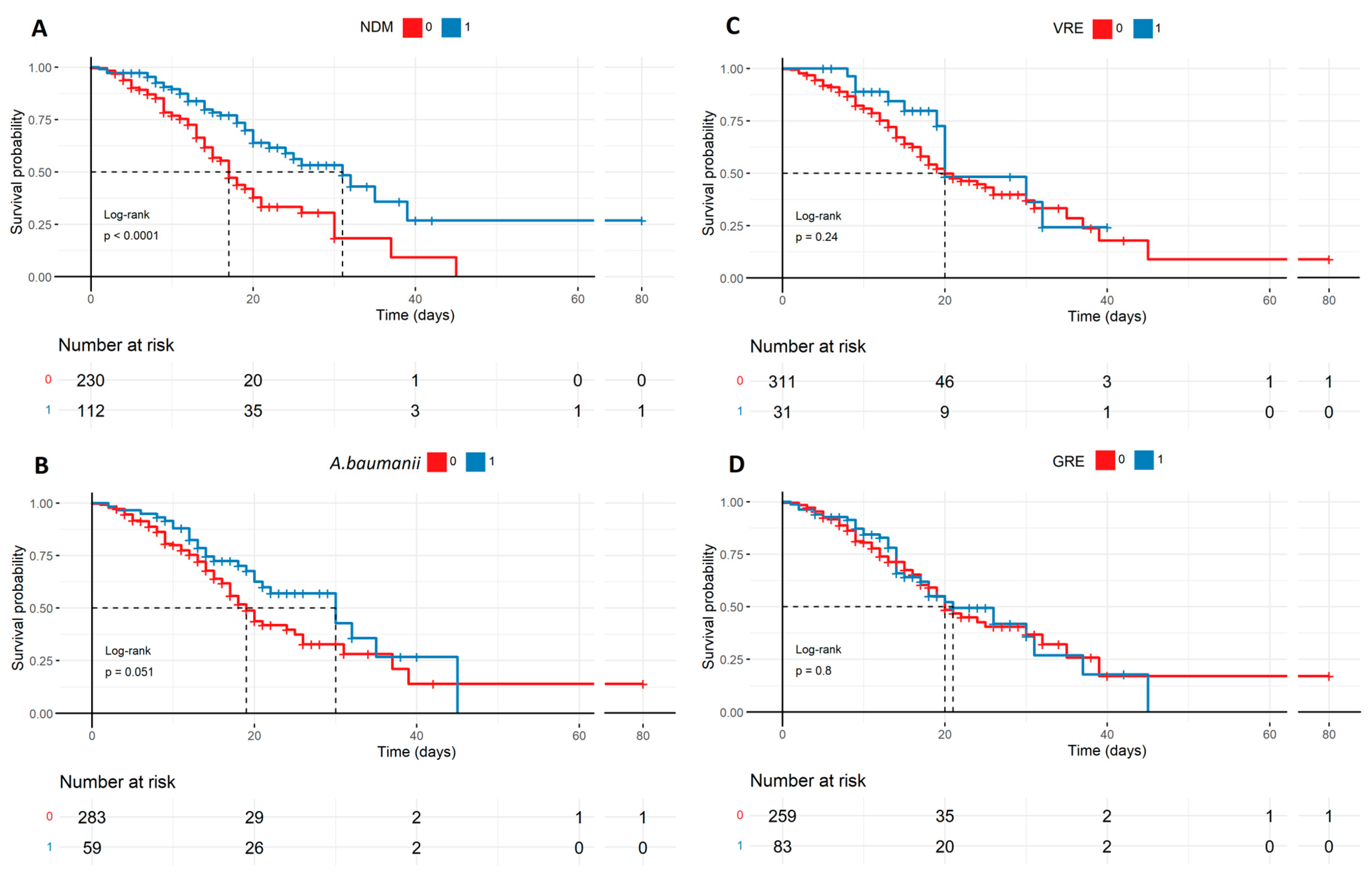
Probability of survival in potential secondary infections with A. baumanii and NDM and the therapy used, described by the Kaplan–Meyer curves. There is a statistical significance in the case of mortality prediction in NDM (B) and A. baumanii (A) in regard to the therapy used (p < 0.009, p < 0.02), favoring patients treated with tocilizumab + steroids and having NDM (+) or A. baumanii (+). On the contrary, patients treated with steroids only, with no secondary infections, were more likely to die. NDM—Klebsiella pneumoniae New Delhi metallo-β-lactamase-resistant, and A. baumanii—Acinetobacter baumanii.
Figure 6.
Probability of survival in potential secondary infections with A. baumanii and NDM and the therapy used, described by the Kaplan–Meyer curves. There is a statistical significance in the case of mortality prediction in NDM (B) and A. baumanii (A) in regard to the therapy used (p < 0.009, p < 0.02), favoring patients treated with tocilizumab + steroids and having NDM (+) or A. baumanii (+). On the contrary, patients treated with steroids only, with no secondary infections, were more likely to die. NDM—Klebsiella pneumoniae New Delhi metallo-β-lactamase-resistant, and A. baumanii—Acinetobacter baumanii.
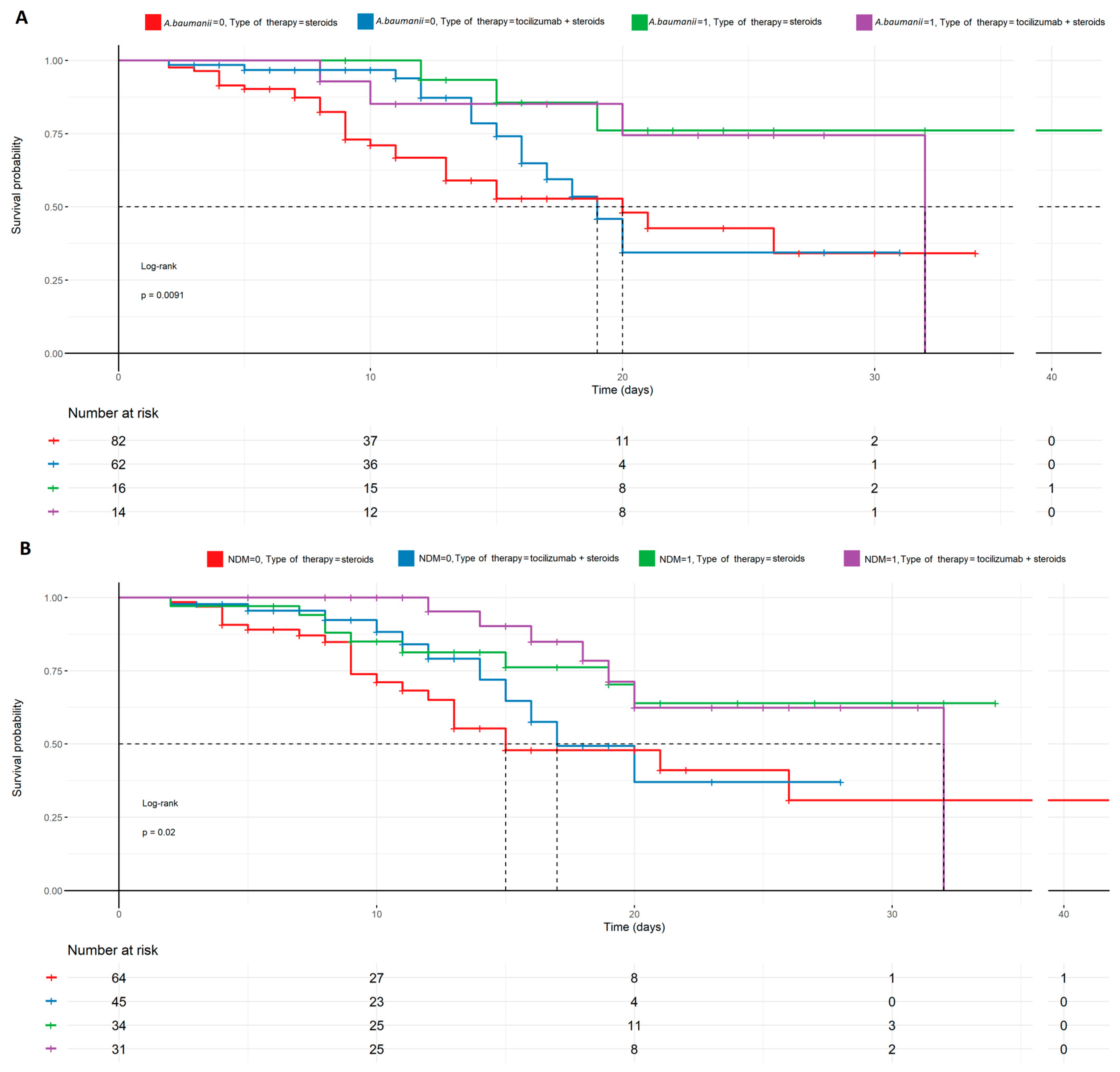
Table 1.
Base model and AIC model.
Table 1.
Base model and AIC model.
Cox Model | AIC Selection Cox Model | |||||
---|---|---|---|---|---|---|
Predictors | HR | CI | p | HR | CI | p |
Baseline characteristics and therapy | ||||||
Sex [Female] | 0.8192 | 0.517–1.2972 | 0.395 | |||
Age (years) [41–60] | 1.5499 | 0.5613–4.2801 | 0.398 | 1.312 | 0.5088–3.3840 | 0.574 |
Age (years) [>60] | 2.7426 | 1.0022–7.5054 | 0.050 | 2.601 | 1.0249–6.5983 | 0.044 |
Tocilizumab + steroids | 0.9417 | 0.5995–1.4793 | 0.794 | |||
Secondary infections and inflammatory parameters | ||||||
NDM | 0.5965 | 0.3840–0.9268 | 0.022 | 0.599 | 0.3910–0.9164 | 0.018 |
GRE | 0.7566 | 0.4829–1.1854 | 0.223 | |||
VRE | 0.7217 | 0.3640–1.4310 | 0.350 | |||
A. baumanii | 0.8889 | 0.5368–1.4720 | 0.647 | |||
PCT | 1.0614 | 1.0107–1.1146 | 0.017 | 1.066 | 1.0193–1.1143 | 0.005 |
CRP | 1.0009 | 0.9990–1.0028 | 0.356 | |||
NEU | 1.0587 | 1.0234–1.0953 | 0.001 | 1.061 | 1.0268–1.0968 | <0.001 |
PLT | 0.9974 | 0.9956–0.9993 | 0.006 | 0.997 | 0.9953–0.9990 | 0.002 |
Comorbidities | ||||||
Obesity | 0.7831 | 0.5204–1.1784 | 0.241 | |||
AH | 1.0401 | 0.6783–1.5950 | 0.857 | |||
AF | 1.0219 | 0.4519–2.3107 | 0.958 | |||
CHD | 1.9252 | 0.8585–4.3175 | 0.112 | 1.778 | 0.9139–3.4582 | 0.090 |
HF | 0.5216 | 0.1386–1.9635 | 0.336 | |||
CKD | 2.1045 | 0.8030–5.5153 | 0.130 | 2.141 | 0.9034–5.0724 | 0.084 |
COPD | 1.3960 | 0.5099–3.8220 | 0.516 | |||
Asthma | 1.7959 | 0.7661–4.2100 | 0.178 | |||
DM2 | 1.3534 | 0.7931–2.3096 | 0.267 | |||
Thyroid diseases | 0.3729 | 0.1424–0.9766 | 0.045 | 0.439 | 0.1830–1.0540 | 0.065 |
Immunosuppression | 2.7168 | 0.3387–21.791 | 0.347 | |||
Cancer | 0.7979 | 0.3021–2.1069 | 0.649 | |||
Autoimmune disease | 1.1245 | 0.4553–2.7775 | 0.799 | |||
Observations | 342 | 342 | ||||
R2 Nagelkerke | 0.205 | 0.182 | ||||
Vaccination status | Unvaccinated 100% |
Disclaimer/Publisher’s Note: The statements, opinions and data contained in all publications are solely those of the individual author(s) and contributor(s) and not of MDPI and/or the editor(s). MDPI and/or the editor(s) disclaim responsibility for any injury to people or property resulting from any ideas, methods, instructions or products referred to in the content. |
Source link
Bartosz Kudliński www.mdpi.com